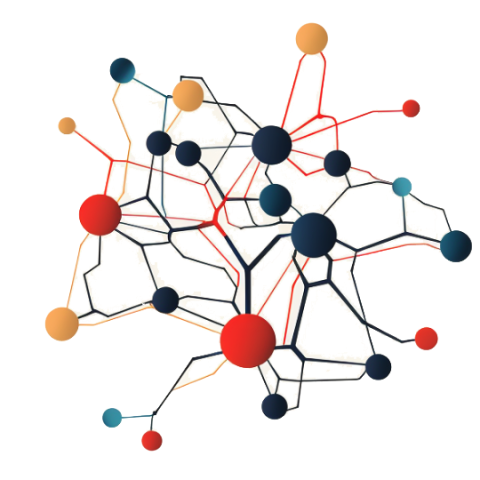
Dynamic AI
Co-Creation
A Human-Centered ApproachCreated through the Digtal Pubishing Initiative at The Creative Media and Digital Culture program, with support of the OER Grants at Washington State University Vancouver.
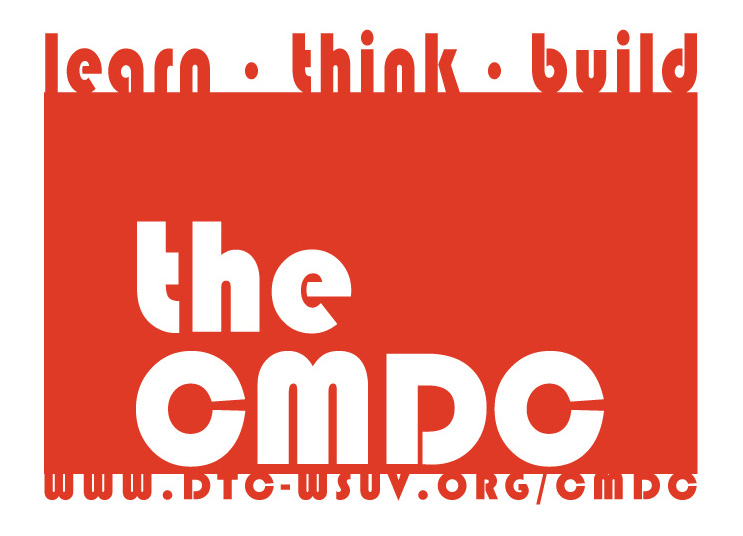
AI image generation tools, such as Midjourney, DALL-E, and Stable Diffusion, have profoundly changed how we can create visual images. These tools synthesize visual works based on text prompts, effectively remixing open source, public domain and copyrighted training data. For instance, a prompt specifying "a Van Gogh landscape painting" creates a dataset of the artists' work online that is then used for analysis and generation of a new image without directly copying any specific painting. But what value does a newly genetrated "Van Gogh" painting have? Such an exercise of copying an artist style provides useful insights into the technology, but is otherwise of little artistic value. On the other hand, a prompt that twists references might be the start of a more interesting creative project. What is "a Van Gogh sculpture?" While AI image generation has raised concerns about copying and copyright infringement, AI image generation extends far beyond mere imitation.
AI tools offer vast potential across numerous fields by providing a powerful means of visual exploration and rapid ideation. For interior designers and architects, AI-generated images can help visualize and refine design concepts, allowing clients to see different styles, color schemes, and layouts before any physical work begins. This can facilitate better communication and decision-making, ensuring that the final design aligns with the client's vision.
Filmmakers can use AI to explore production elements such as location, costume, and lighting ideas before committing significant resources. By generating visual mock-ups, directors can experiment with different atmospheres and aesthetics, aiding in pre-visualization and enhancing creative planning. This iterative process can lead to more informed and effective production decisions, ultimately saving time and money.
In education, AI-generated images can be used to create engaging and illustrative materials for teaching complex concepts. In research, scientists might visualize data or create conceptual visualizations in novel ways, enabling new insights and discoveries. Artists can collaborate with AI to push the boundaries of their creativity, exploring unique styles and compositions that might not be possible through traditional means.
AI image generation tools have catalyzed numerous legal and ethical quandaries around copyright ownership and the nature of intellectual property in the age of machine intelligence. Some argue that the transformative process of an AI generating a new image from semantic prompts constitutes fair use. Others contend that commercial interests driving AI companies necessitate licensing agreements with rights holders for any training data usage. Beyond copyright, there are philosophical questions about whether AI-generated images can be granted copyright protections, as they are computational outputs lacking a clear human author. There are also concerns about generative AI's potential for enabling new forms of misinformation and manipulation, such as deepfake images and videos.
As generative AI becomes more ubiquitous across creative industries, there is a need for an evolution in legal frameworks to address these issues. At the same time, there is great cultural value in exploring the creative possibilities of these tools in ethical and human-centered ways.
Generative Adversarial Networks (GANs) are a type of AI used to create images. Central to how GANs work is something called latent space. You can think of latent space as a hidden map, where each point represents the potential for creating a unique image.The generator in a GAN is like an artist that takes a set of coordinates (called a latent vector) from this map and transforms them into an image based on what it has learned from existing pictures.
As the generator improves over time, it gets better at turning these coordinates into more realistic images. The discriminator acts like an art critic, judging the results and helping the generator refine its skills.
In simpler terms, latent space is where the GAN explores different possibilities. By moving through this space, the AI can create new and varied images that resemble the data it has been trained on. The closer two points are in latent space, the more similar the images they produce will be.
Generative Adversarial Networks (GANs) work through a back-and-forth process between two parts: one that creates images and one that evaluates them. This interaction leads to a kind of "synthetic imagination," where the AI generates new visuals that imitate the styles and details of the images it was trained on but with unique variations. Even small changes in the input can produce very different results, balancing between order and creativity. However, GANs do have limitations, such as mode collapse (when the AI keeps generating the same or similar images) and training instability (when the learning process breaks down), which can cause issues like repeated patterns or flawed images.
Diffusion models, an alternative to GANs, are another powerful method for generating AI images. They operate quite differently: instead of a generator and discriminator working in tandem, diffusion models start with random noise and iteratively "denoise" it into a coherent image. The process is inspired by how particles diffuse in physics but reversed—beginning with noise and gradually refining it until a high-quality image emerges. Essentially, diffusion models learn to predict and remove the noise step-by-step, guided by the patterns found in their training data. This makes them particularly effective at creating highly detailed, photorealistic images.
Diffusion models excel at flexibility and stability during training, as they don’t rely on the adversarial setup of GANs, which can lead to issues like mode collapse. Moreover, diffusion models can often create more diverse outputs without falling into repetitive patterns. They are the technology behind popular tools like DALL-E 2, Stable Diffusion, and Google’s Imagen, making them a central force in AI image generation today.
While GANs and diffusion models each have unique strengths, the current trend suggests a growing preference for diffusion models in many applications. This shift is largely due to their stability, ability to generate diverse results, and suitability for generating fine details. GANs remain useful in certain contexts, especially when computational speed is critical, but their limitations in training stability and diversity of output often make them less versatile.
Looking ahead, hybrid approaches that combine the strengths of both GANs and diffusion models may emerge, optimizing for both quality and efficiency. Additionally, diffusion models are already influencing fields like video generation, where their iterative refinement process aligns well with the complexities of motion and texture. As AI continues to evolve, diffusion-based techniques seem poised to become the foundation for next-generation creative tools, offering artists and creators unprecedented levels of control, quality, and innovation.
The first AI artwork to be sold at auction, Edmond de Belamy, marked a groundbreaking moment in the art world, showcasing the novel capabilities of machine-generated creativity. Created by the Paris-based art collective Obvious, this portrait was not painted by a human hand but by a machine. Utilizing a Generative Adversarial Network (GAN), the AI was trained on a dataset of 15,000 portraits spanning six centuries. The resulting piece, characterized by its hauntingly abstract features, reflects the essence of classic portraiture while simultaneously challenging traditional notions of artistry. When Edmond de Belamy was auctioned at Christie’s in 2018, it fetched an astonishing $432,500, far exceeding initial estimates. This sale highlighted the profound potential of AI in art, demonstrating that, with the right data and algorithms, machines can produce works that are both innovative and evocative, without direct human intervention beyond their initial programming.
While GANs and diffusion models are powerful visual creation tools, many artists use AI as a creative assistant rather than an autonomous image generator. Key strategies include:
These methods emphasize the human's central creative role in priming, curating, and combining the AI's generative capabilities with their own aesthetics and visions. AI becomes a collaborator and tutor, expanding the toolset for ideation and realization while respecting the artist's orchestration of the final artifacts.
The past few years have witnessed an explosive proliferation of platforms and software tools enabling AI-assisted image creation and manipulation:
The rapid pace of development in this space means that incredibly capable new tools are emerging constantly. With many offered through accessible web interfaces or applications, AI-powered visual creation is quickly becoming open to everyone, not just skilled artists and developers.
While the AI art tools themselves are impressive technological marvels, equally essential are the pioneering human artists pushing the boundaries of how this tech can expand modes of creative expression and communication. Notable AI artists include:
Sofia Crespo is a pioneering artist whose work focuses on the intersection of biology and artificial intelligence. She uses AI models, particularly GANs and neural networks, to create intricate digital art pieces that explore the relationship between natural and artificial life forms. Her work often features organic shapes and textures reminiscent of biological entities, reflecting on how AI can mimic and interpret the complexities of the natural world. Crespo’s notable projects include "Neural Zoo," where she generates images of speculative creatures and plants that do not exist in reality but appear convincingly organic, challenging our perceptions of nature and machine-generated art.
Sougwen Chung is an interdisciplinary artist who explores the relationship between humans and machines through collaborative drawings and installations. Her work frequently involves drawing alongside robotic arms controlled by AI systems that learn from her style. This ongoing human-AI collaboration, as seen in her project "Drawing Operations," highlights the evolving nature of co-creativity and questions the role of autonomy in artistic creation. Chung’s practice bridges art, performance, and technology, offering a poetic exploration of how humans and machines can create together.
Refik Anadol is an artist known for his immersive installations that transform data into visually stunning and thought-provoking art pieces. He leverages AI and machine learning algorithms to process large datasets, such as urban landscapes, social media interactions, and cultural archives, turning them into dynamic visualizations and media sculptures. Anadol's work often involves projecting these data-driven visuals onto architectural surfaces, creating a seamless blend of the physical and digital realms. His projects like "Infinity Room" and "Melting Memories" push the boundaries of how data can be experienced aesthetically, offering a glimpse into the future of media art and the potential of AI to reshape our interaction with information.
Anna Ridler is an artist who works with both AI and data to explore themes of narrative, storytelling, and ownership of information. She often creates hand-annotated datasets, which are then used to train AI models, allowing her to maintain creative control over the outputs. One of her most well-known works is "Mosaic Virus," where she used a dataset of tulip images to comment on historical tulip mania and contemporary financial bubbles. Ridler’s work offers a thoughtful examination of how humans can shape the datasets that AI learns from, turning the creative process into a more personal and intentional act.
Ben Snell is an artist who uses artificial intelligence to explore the intersection of human creativity and machine learning. He trains custom AI models on datasets of his own sculptures, letting the machine generate new forms that he then brings into physical existence. By transforming AI-generated concepts into tangible objects, Snell's work investigates the evolving relationship between creator and tool, and the ways in which machines can co-author the artistic process. His project "Dio" involved feeding a computer's physical body back into the machine, creating a self-referential loop of artistic creation.
Roman Lipski is a Polish painter who collaborates with artificial intelligence to push the boundaries of his artistic practice. Through a constant exchange between the artist and an AI system, Lipski's work has evolved into a process of co-creation where the machine suggests novel ideas based on his past works. His "Unfinished" series is an ongoing dialogue between human intuition and machine learning, where AI not only inspires but also influences the creative process. This partnership explores the potential of AI to expand human imagination and challenges traditional notions of authorship in the arts.
This exercise explores how comic artists can leverage AI image generation tools to enhance their creative workflow, from initial sketches to final colored panels: