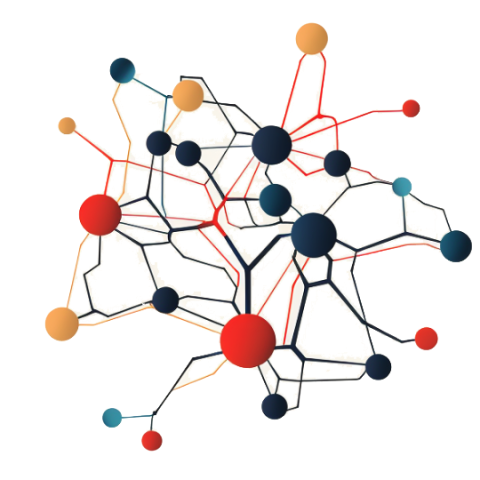
Dynamic AI
Co-Creation
A Human-Centered ApproachCreated through the Digtal Pubishing Initiative at The Creative Media and Digital Culture program, with support of the OER Grants at Washington State University Vancouver.
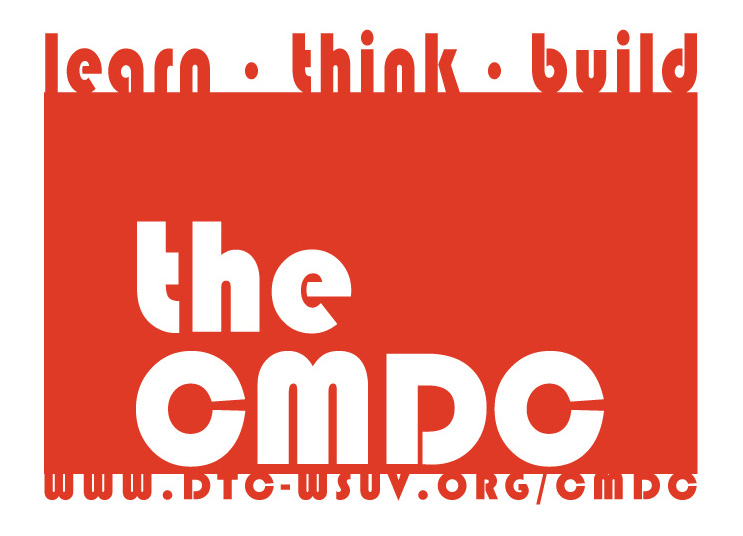
Pioneers like Lejaren Hiller and Leonard Isaacson, with their 1957 Illiac Suite, demonstrated the early potential of computers in music composition. Today, modern tools like Google's Magenta and OpenAI's MuseNet build on this legacy, generating polyphonic compositions that challenge the conventional boundaries of music. These tools provide a starting point for human creators, who can use iterative processes to refine and shape AI-generated music, voice, and sound effects into works that reflect their personal artistic vision.
AI generative music represents a profound intersection of technology and creativity, where machines collaborate with human artists to push the boundaries of what is sonically possible. The complexity and historical challenges of computer-generated music stem from the need to understand and replicate the intricate aspects of musical composition, including harmony, rhythm, and structure. To generate coherent and compelling music, AI models must be capable of producing long sequences of audio that maintain temporal, spectral complexity, harmony, rhythm and structure.
Achieving coherence over long sequences of music is particularly challenging. AI must balance repetition and variation to avoid creating music that is either monotonous or disjointed. This requires sophisticated models.
Together, these advanced AI models—CNNs, RNNs, LSTMs, and GANs—work in concert to address the inherent complexities of music generation. They allow AI to produce not just individual notes or short loops, but entire compositions that evolve naturally over time, demonstrating the potential of AI to not only mimic human creativity but to extend it in new and innovative ways.
The evolution of AI voice synthesis has been a remarkable journey, from the mechanical speech synthesis of the early 20th century to the seamless mimicry of human speech achieved by modern models like Eleven Labs and HeyGen Labs. Early efforts, such as the Voder demonstrated at the 1939 World's Fair, relied on manual control of a keyboard and foot pedals to produce speech sounds. While innovative, these early systems were limited in expressiveness and naturalness.
In the 1950s and 1960s, formant synthesis emerged, using knowledge-based algorithms to simulate the resonant frequencies of the human vocal tract. Although this approach represented a significant advancement, the resulting speech often sounded robotic. The 1990s saw the rise of concatenative synthesis, which involved stitching together small units of recorded speech. This method greatly improved naturalness but still struggled with intonation and emotion.
Recent advances in deep learning have revolutionized AI voice generation. Techniques like WaveNet, introduced by DeepMind in 2016, leverage Convolutional Neural Networks (CNNs) and Recurrent Neural Networks (RNNs), including Long Short-Term Memory (LSTM) networks, to produce speech that closely mimics the human voice in terms of naturalness, intonation, and emotion. These models are capable of generating highly realistic and expressive synthetic voices that can be tailored to a wide range of applications, from audiobook narration and virtual assistants to video game character voices.
However, the powerful capabilities of AI voice modeling come with significant challenges and risks. One of the primary challenges is achieving naturalness and expressiveness in synthesized speech, which involves capturing human emotions, tone, and style in a way that feels authentic. Additionally, there is the need for language and accent diversity, which requires extensive datasets and sophisticated modeling to ensure inclusivity and accuracy across a vast array of dialects and languages. Computational demands also pose a challenge, as high-quality voice synthesis requires substantial resources, making real-time processing with minimal latency difficult to achieve on low-power devices.
Another critical aspect is personalization and adaptability, where AI systems must be capable of adapting to individual voices and speaking styles to create personalized speech generation. Despite these challenges, the benefits of AI voice synthesis are profound, particularly in enhancing accessibility. AI-generated voices can provide high-quality audio readings of text for visually impaired individuals, offering greater access to information and literature. These tools can also assist those who have difficulty reading due to dyslexia or other learning disabilities by converting written content into easily understandable audio.
Beyond accessibility, AI voice technology has the potential to revolutionize education by enabling the creation of personalized and engaging learning materials. It can enhance user experiences in customer service, entertainment, and communication by providing natural-sounding, responsive, and emotionally expressive voices in various applications. As AI voice synthesis continues to advance, it is essential to balance its powerful capabilities with considerations of ethical use, ensuring that the technology serves to enhance human experience while safeguarding against potential harms. The potential for misuse, such as creating deepfakes or impersonating individuals, underscores the need for responsible use and ethical guidelines in the development and deployment of AI voice technologies.
AI is not only transforming music and voice synthesis but also expanding the possibilities of sound effects. The ability to generate rich and immersive soundscapes is vital for enhancing interactive experiences across various media, from video games and virtual reality to films and multimedia installations.
Generative models trained on vast libraries of sound effects can create realistic and context-sensitive audio environments such as the rustling of leaves in a virtual forest or the bustling streets of a digital metropolis. These AI-driven sound effects are integral to storytelling, allowing creators to craft immersive and believable worlds that engage the audience's senses on a deeper level.
Moreover, AI sound effect generation can be tailored to specific needs, enabling the creation of unique and novel sound palettes that push the boundaries of traditional sound design. This versatility makes AI an invaluable tool for industries ranging from gaming and film to advertising and interactive installations, where compelling audio experiences are crucial for engaging audiences.
The rise of generative audio tools such as Stable Audio, Boomy, and Suno.ai has democratized the field of sound production, empowering creators with unprecedented ease and accessibility. These platforms harness the power of AI to offer innovative tools for composing, sculpting, and manipulating soundscapes, breaking down barriers that once restricted access to professional-grade audio production.
Stable Audio, for instance, leverages advanced generative models to create a wide range of audio content, from music and sound effects to ambiances and foley. Creators can provide textual descriptions or seed audio samples, and the AI will generate complex and nuanced audio sequences tailored to their needs.
Similarly, Boomy and Suno.ai offer intuitive interfaces that allow users to explore and manipulate audio in ways that were previously unimaginable. These tools enable creators to experiment with sound design, remixing, and audio manipulation, empowering them to bring their artistic visions to life with unprecedented freedom and creativity.
The democratization of audio production through AI-powered tools has opened up new avenues for expression, fostering a vibrant ecosystem of audio creators from professionals to hobbyists and paving the way for innovative collaborations between human artists and artificial intelligence.
AI's influence on the music industry extends far beyond composition, touching upon various aspects of the creative process and challenging the traditional roles and boundaries within the industry. From AI-collaborated albums like Taryn Southern's "I AM AI" and Björk's "Kórsafn" to real-time interactive performances, AI is redefining the dynamics of musical creation and expression.
In music composition, AI serves as a powerful co-creator, offering suggestions and inspiration that can augment the musician's toolkit and unlock new realms of artistic exploration. This collaborative dynamic blurs the lines between the artist and the tool, inviting a reexamination of the creative process itself.
Beyond composition, AI is also making significant inroads in music analysis, processing, and real-time interaction. Advanced algorithms can analyze and deconstruct existing music, providing insights into structure, harmony, and emotion, enabling musicians to refine and enhance their craft. Additionally, AI-powered tools for audio processing and mastering offer new levels of precision and control, allowing artists to shape their sonic visions with unprecedented clarity.
In live performances, AI opens up exciting possibilities for real-time interaction and improvisation. Generative models can respond to and adapt to the performers' inputs, creating a symbiotic relationship between the human artist and the artificial intelligence, resulting in truly unique and dynamic musical experiences.
As AI continues to permeate the music industry, it invites a reevaluation of the roles and boundaries within the creative process, fostering a collaborative environment where human artistry and technological innovation converge to push the boundaries of musical expression.
In this exercise, students will explore the creative possibilities of AI in audio production by creating an original soundtrack or audio narrative that includes music, voice, and sound effects. The focus will be on guiding the AI to produce non-traditional, imaginative music and/or soundscapes rather than simply mimicking human-created audio.
Create a fictional band from scratch using ChatGPT and various songs of the band's fictional album using AI Audio tools. Develop the band's identity, including their name, member names, backgrounds, and stylistic influences in a Chat session with ChatGpt. Then generate a few tracks for the band and generate corresponding images for each song.
The discourse surrounding AI's impact on audio creation touches upon a wide range of thought-provoking topics, inviting deeper contemplation of the relationship between human artistry and technological innovation. Key discussion questions include: